Flower A Friendly Federated Learning Framework
A unified approach to federated learning, analytics, and evaluation. Federate any workload, any ML framework, and any programming language.
to learn federated learning
Flower Monthly: 7th Aug 17:00 GMTThe next Flower Monthly is scheduled for 7th Aug 16:00 UTC (09:00 SF, 12:00 NY, 17:00 LON, 18:00 CET, 21:30 IST, 00:00 北京)
Users love Flower
Some of the best organizations in the world use Flower
Get Started
Getting started with Flower can be so easy. Code examples show different usage scenarios of Flower in combination with popular machine learning frameworks.
0. Install Flower and TensorFlow
pip install flwr tensorflow
1. client.py
import flwr as fl
import tensorflow as tf
# Load model and data (MobileNetV2, CIFAR-10)
model = tf.keras.applications.MobileNetV2((32, 32, 3), classes=10, weights=None)
model.compile("adam", "sparse_categorical_crossentropy", metrics=["accuracy"])
(x_train, y_train), (x_test, y_test) = tf.keras.datasets.cifar10.load_data()
# Define Flower client
class CifarClient(fl.client.NumPyClient):
def get_parameters(self, config):
return model.get_weights()
def fit(self, parameters, config):
model.set_weights(parameters)
model.fit(x_train, y_train, epochs=1, batch_size=32)
return model.get_weights(), len(x_train), {}
def evaluate(self, parameters, config):
model.set_weights(parameters)
loss, accuracy = model.evaluate(x_test, y_test)
return loss, len(x_test), {"accuracy": accuracy}
# Start Flower client
fl.client.start_numpy_client(server_address="127.0.0.1:8080", client=CifarClient())
2. server.py
import flwr as fl
# Start Flower server
fl.server.start_server(
server_address="0.0.0.0:8080",
config=fl.server.ServerConfig(num_rounds=3),
)
Federated Learning Tutorials
This series of tutorials introduces the fundamentals of Federated Learning and how to implement it with Flower.
00
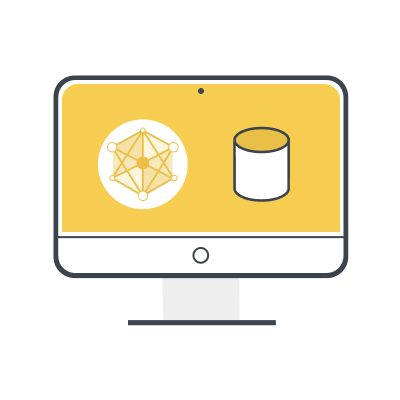
What is Federated Learning?
- Classic Machine Learning
- Challenges of Classical Machine Learning
- Federated Learning
- Federated Evaluation
- Federated Analytics
- Differential Privacy
01
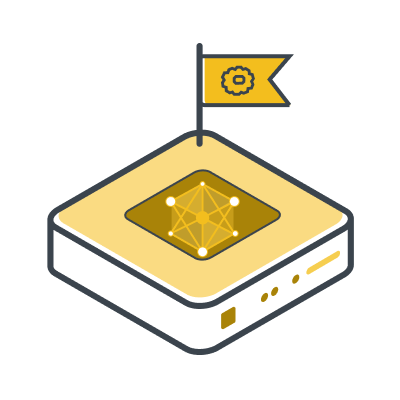
Get started with Flower
- Preparation
- Step 01: Centralized Training with PyTorch
- Step 02: Federated Learning with Flower
Check out the rest of the tutorials:
Getting Started
Installation Guide
The Flower documentation has detailed instructions on what you need to install Flower and how you install it. Spoiler alert: you only need pip! Check out our installation guide.
_Why Flower?
A unified approach to federated learning, analytics, and evaluation.
Scalability
Flower was built to enable real-world systems with a large number of clients. Researchers used Flower to run workloads with tens of millions of clients.
ML Framework Agnostic
Flower is compatible with most existing and future machine learning frameworks. You love Keras? Great. You prefer PyTorch? Awesome. Raw NumPy, no automatic differentiation? You rock!
Cloud, Mobile, Edge & Beyond
Flower enables research on all kinds of servers and devices, including mobile. AWS, GCP, Azure, Android, iOS, Raspberry Pi, and Nvidia Jetson are all compatible with Flower.
Research to Production
Flower enables ideas to start as research projects and then gradually move towards production deployment with low engineering effort and proven infrastructure.
Platform Independent
Flower is interoperable with different operating systems and hardware platforms to work well in heterogeneous edge device environments.
Usability
It's easy to get started. 20 lines of Python is enough to build a full federated learning system. Check the code examples to get started with your favorite framework.
Join theCommunity!
Join us on our journey to make federated approaches available to everyone.