Share this post
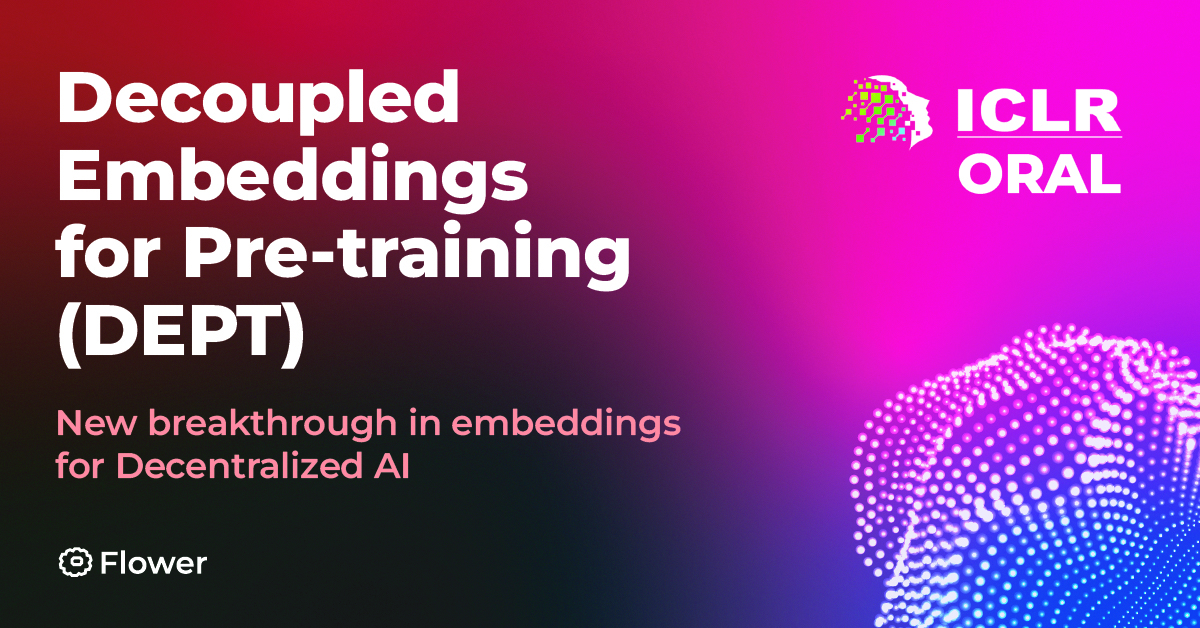
We are excited to share a new approach to large-scale language model pre-training — Decoupled Embeddings for Pre-training (DEPT) — which has been accepted as an Oral at ICLR 2025 in Singapore. DEPT tackles a fundamental challenge in language modeling: integrating diverse datasets spanning different domains, languages, and styles. DEPT will be presented at ICLR next Saturday, April 26.
The Challenge: Negative Interference and the Curse of Multilinguality
As language model training scales to ever-broader corpora, we encounter two persistent issues:
- Negative Interference: When diverse data sources (e.g., code, biomedical text, casual dialogue) vie for limited model capacity, each domain can degrade the learning outcomes of the others.
- Curse of Multilinguality: Adding more languages boosts coverage but yields diminishing returns— especially acute for low-resource languages. Standard solutions (e.g., multilingual BERT, XLM, mT5, and large LLMs like LLaMA) rely on globally shared embeddings, leading to vocabulary dilution and sub-optimal cross-lingual/domain performance. They often require painstaking temperature-tuning of language sampling ratios or heuristics for domain weighting, adding overhead and complexity.
Our Proposal: DEPT
DEPT is a communication-efficient pre-training framework that bridges multiple data sources without relying on a single shared vocabulary or embeddings. The core concept is to decouple token embeddings from the transformer body, optimizing them separately for each data source while maintaining a shared core model. This yields the following benefits:
- Reduced Memory Footprint By only storing and exchanging the embedding parameters needed for each data silo, DEPT cuts the embedding matrix size substantially—often saving multiple hundreds of millions of parameters.
- Fewer Communication Bottlenecks DEPT’s focus on lightweight parameter averaging significantly reduces the data exchanged across distributed clients. By shrinking embedding layers, it directly cuts communication overhead in proportion to the parameter reduction and update frequency.
- Improved Robustness and Plasticity DEPT-trained transformers handle data heterogeneity more gracefully, showing stronger generalization to new domains and languages. The transformer body concentrates on learning abstract, high-level features, while local embeddings capture domain- or language-specific nuances.
- Vocabulary-Agnostic Federated Training DEPT can train billion-scale LMs across silos that each have unique tokenizers and vocabularies, demonstrating vocabulary-agnostic federated pre-training. Practitioners can thus add new data sources without forcing a global tokenizer across every domain.
Three DEPT Variants
We have developed three distinct DEPT variants that offer a variety of performance enhancements and implementation options.
- GLOB: Maintains a single global embedding matrix but employs local updates to reduce synchronization overhead.
- TRIM: Allows each data silo to “trim” unused vocabulary entries, preventing them from needlessly bloating the embedding matrix or communication traffic.
- SPEC: Fully decouples embeddings for each data silo, enabling true vocabulary-agnostic pre-training. This approach needs a final global embedding stage but greatly reduces per-round communication.
DEPT: Significant Performance Wins
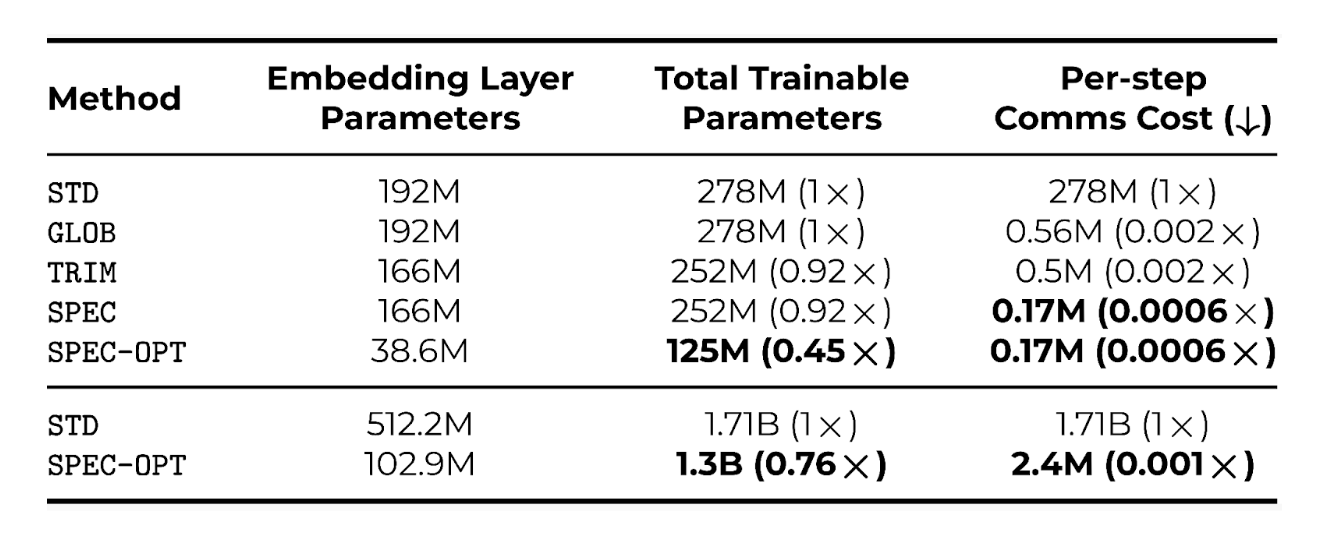
Table 1. Memory and communication costs for multilingual models trained with the Standard (STD) method vs GLOB, TRIM, SPEC. SPEC-OPT refers to a version of SPEC with an optimized local vocabulary. Columns show, in order, the method name, the dimension of the embedding layer in millions of parameters, the total size/memory requirements of the model in millions of parameters, and the amount of data each worker sends and receives each round. DEPT variants bring considerable improvements in all shown metrics.
This exciting new methodology has several practical advantages and an impressive performance on a set of pre-training scenarios we used to evaluate it.
- Effective Reduction of Memory Footprint The DEPT approach to decoupled embeddings allowed us to train multilingual models while reducing their embedding matrix size by 80% and reducing total necessary VRAM by up to 24% using SPEC compared to the standard approach, as shown in the final two rows of Table 1, showcasing our billion-scale model. Freeing up the memory allows DEPT to use larger batch sizes.
- Massive Communication Costs Reduction All variants of DEPT leverage communication efficient optimization based on LocalSGD, as shown in Table 1. The GLOB variant shows that this alone can reduce communication costs by up to 500x. Using TRIM, costs are further reduced due to the thoughtful manipulation of the embedding layer, bringing total savings up to 560x for our multilingual setting on top of the gains from GLOB. The most savings appear when using the SPEC variant, which doesn’t require exchanging the often heavy embedding layers. SPEC can save 714x communication costs compared to the standard approach when training billion-scale models.
- Competitive Downstream Performance The most interesting result every practitioner would like to see regards the downstream performance of DEPT models. Despite all the cost reduction that DEPT can bring, our models can effectively compete with those trained using the standard approach. In order to provide a fair comparison between the specialized embeddings of SPEC and those of the standard baseline, we evaluate all models after continued pre-training + downstream fine-tuning, starting with a randomly initialized embedding matrix. As shown in Table.2, we obtain improvements up to 4.1%-7.5% in the final downstream performance metric over the baselines, with DEPT variants outperforming most of the time.
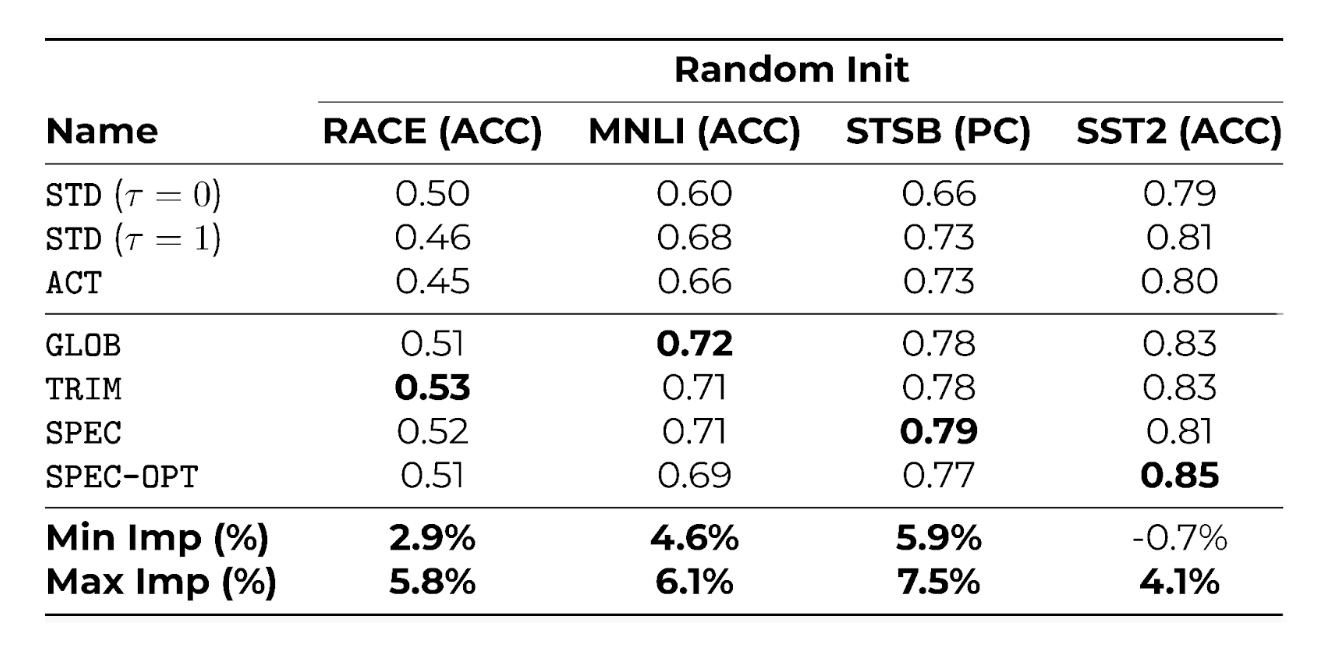
Table 2. Downstream task performance after continued pre-training starting from a random initialization + downstream fine-tuning. The metrics shown are Accuracy (ACC) and Pearson Correlation (PC) on the standard RACE, MNLI, STSB, and SST2 benchmarks.
For further details on the methodology and the empirical evaluation, read our paper and feel free to reach out to ask any questions.
Broader Impact: A New Paradigm
for Pre-training
We envision DEPT as the foundation of a new paradigm for pre-training language models. By embracing decoupled embeddings on heterogeneous data, it produces a more generalizable and adaptive transformer body than standard methods. Concretely, the implications are:
- Flexibility: Practitioners can train on highly diverse and even private data sources without the complexities of designing and maintaining a single, unified vocabulary.
- Efficiency: Substantially lower communication and memory costs let organizations pre-train across geographically distributed GPUs, enabling large-scale, low-bandwidth deployment.
- Generality: DEPT-trained models can serve as versatile foundation models that perform well across multiple tasks and domains. Compared to standard pre-training, such models often enhance the efficacy of continued training and fine-tuning pipelines. This approach not only simplifies training setups but also extends the applicability of pre-trained models to settings where data is diverse, distributed, or subject to strict privacy constraints.
Key Takeaways and Future Directions
DEPT’s decoupling strategy has shown:
- Significant memory savings in embedding layers, especially important for multilingual or domain-heavy LMs.
- Better resilience to heterogeneous data, mitigating negative interference.
- Scalability to billions of parameters, with dramatic communication savings compared to standard data-parallel training. Moving forward, we aim to expand DEPT’s capabilities to support:
- More flexible embedding-generation methods (especially for SPEC), such as zero-shot embedding transfer, vocabulary matching, or model stitching.
- Larger domain coverage while preserving model quality, enabling broader adoption for low-resource languages and specialized data domains.
Stay tuned for more details on Decoupled Embeddings for Pre-training (DEPT) as we present our work at ICLR 2025 in Singapore. We look forward to seeing how researchers and practitioners adopt and extend this approach to build next-generation language models across highly diverse and distributed data sources.
Share this post