Exploiting Shared Representations for Personalized Federated Learning¶
Paper: arxiv.org/abs/2102.07078
Authors: Liam Collins, Hamed Hassani, Aryan Mokhtari, Sanjay Shakkottai
Abstract: Deep neural networks have shown the ability to extract universal feature representations from data such as images and text that have been useful for a variety of learning tasks. However, the fruits of representation learning have yet to be fully-realized in federated settings. Although data in federated settings is often non-i.i.d. across clients, the success of centralized deep learning suggests that data often shares a global feature representation, while the statistical heterogeneity across clients or tasks is concentrated in the labels. Based on this intuition, we propose a novel federated learning framework and algorithm for learning a shared data representation across clients and unique local heads for each client. Our algorithm harnesses the distributed computational power across clients to perform many local-updates with respect to the low-dimensional local parameters for every update of the representation. We prove that this method obtains linear convergence to the ground-truth representation with near-optimal sample complexity in a linear setting, demonstrating that it can efficiently reduce the problem dimension for each client. This result is of interest beyond federated learning to a broad class of problems in which we aim to learn a shared low-dimensional representation among data distributions, for example in meta-learning and multi-task learning. Further, extensive experimental results show the empirical improvement of our method over alternative personalized federated learning approaches in federated environments with heterogeneous data.
About this baseline¶
What’s implemented: The code in this directory replicates the experiments in Exploiting Shared Representations for Personalized Federated Learning (Liam Collins et al., 2021) for CIFAR10 and CIFAR-100 datasets, which proposed the FedRep
model. Specifically, it replicates the results of CIFAR-10 ((100, 2), (100, 5)
) and CIFAR-100 ((100, 5), (100, 20)
) found in table 1 in their paper.
Datasets: CIFAR-10, CIFAR-100 from Flower Datasets
.
Hardware Setup: WSL2 Ubuntu 22.04 LTS, NVIDIA RTX 3070 Laptop, 32GB RAM, AMD Ryzen 9 5900HX.
Contributors: Jiahao Tan<karhoutam@qq.com>
Experimental Setup¶
Task: Image Classification
Model: This directory implements 2 models:
CNNCifar10
CNNCifar100
These two models are modified from the official repo’s. To be clear that, in the official models, there is no BN layers. However, without BN layer helping, training will definitely collapse.
Please see how models are implemented using a so called model_manager and model_split class since FedRep uses head and base layers in a neural network. These classes are defined in the models.py
file. Please, extend and add new models as you wish.
Dataset: CIFAR10, CIFAR-100. CIFAR10/100 will be partitioned based on number of classes for data that each client shall receive e.g. 4 allocated classes could be [1, 3, 5, 9].
Training Hyperparameters: The hyperparameters can be found in pyproject.toml
file under the [tool.flwr.app.config]
section.
Description |
Default Value |
---|---|
|
|
|
|
|
|
|
|
|
|
|
|
|
|
|
|
|
|
|
|
|
|
|
|
Environment Setup¶
Create a new Python environment using pyenv and virtualenv plugin, then install the baseline project:
# Create the environment
pyenv virtualenv 3.10.12 fedrep-env
# Activate it
pyenv activate fedrep-env
# Then install the project
pip install -e .
Running the Experiments¶
flwr run . # this will run using the default settings in the `pyproject.toml`
While the config files contain a large number of settings, the ones below are the main ones you’d likely want to modify.
algorithm = "fedavg", "fedrep" # these are currently supported
dataset-name = "cifar10", "cifar100"
dataset-split-num-classes = 2, 5, 20 (only for CIFAR-100)
model-name = "cnncifar10", "cnncifar100"
See also for instance the configuration files for CIFAR10 and CIFAR100 under the conf
directory.
Expected Results¶
The default algorithm used by all configuration files is fedrep
. To use fedavg
please change the algorithm
property in the respective configuration file. The default federated environment consists of 100 clients.
When the execution completes, a new directory results
will be created with a json file that contains the running configurations and the results per round.
[!NOTE] All plots shown below are generated using the
docs/make_plots.py
script. The script reads all json files generated by the baseline inside theresults
directory.
CIFAR-10 (100, 2)¶
flwr run . --run-config conf/cifar10_2.toml
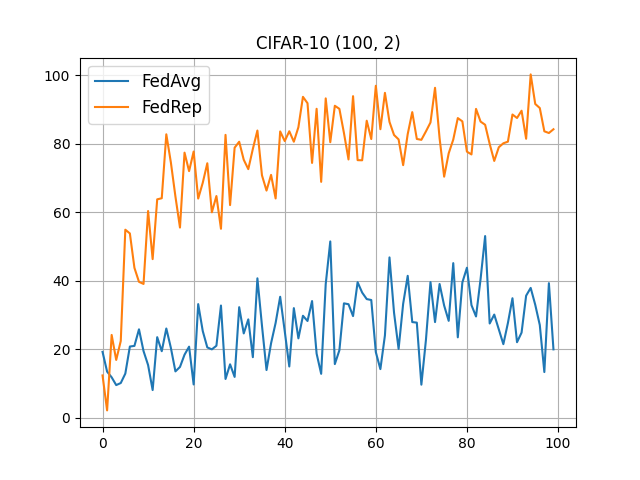
CIFAR-10 (100, 5)¶
flwr run . --run-config conf/cifar10_5.toml
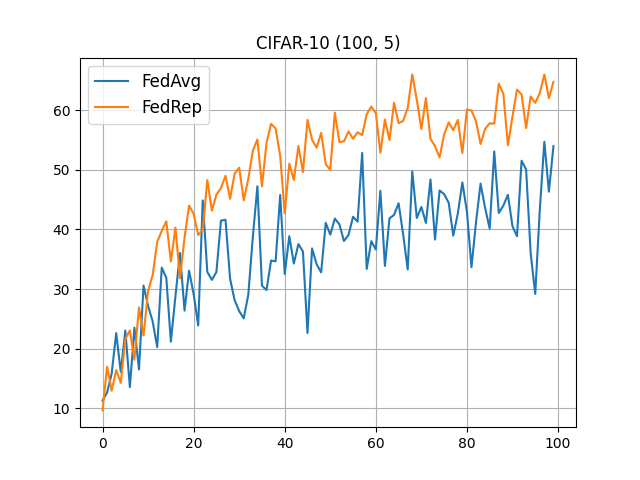
CIFAR-100 (100, 5)¶
flwr run . --run-config conf/cifar100_5.toml
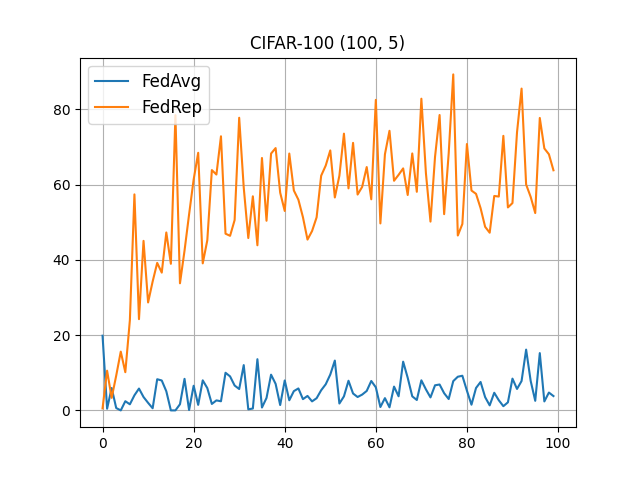
CIFAR-100 (100, 20)¶
flwr run . --run-config conf/cifar100_20.toml
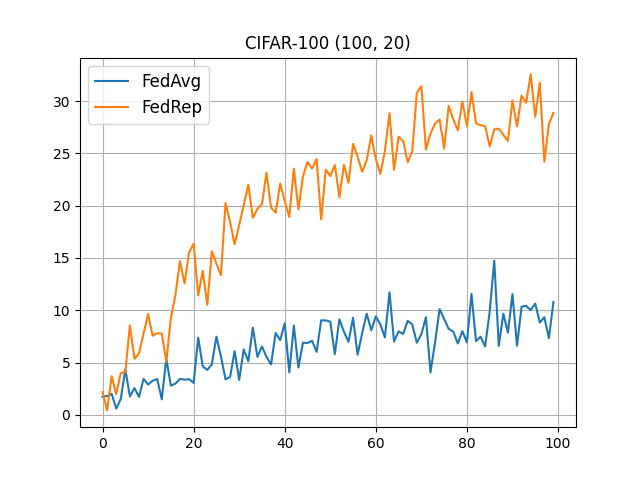