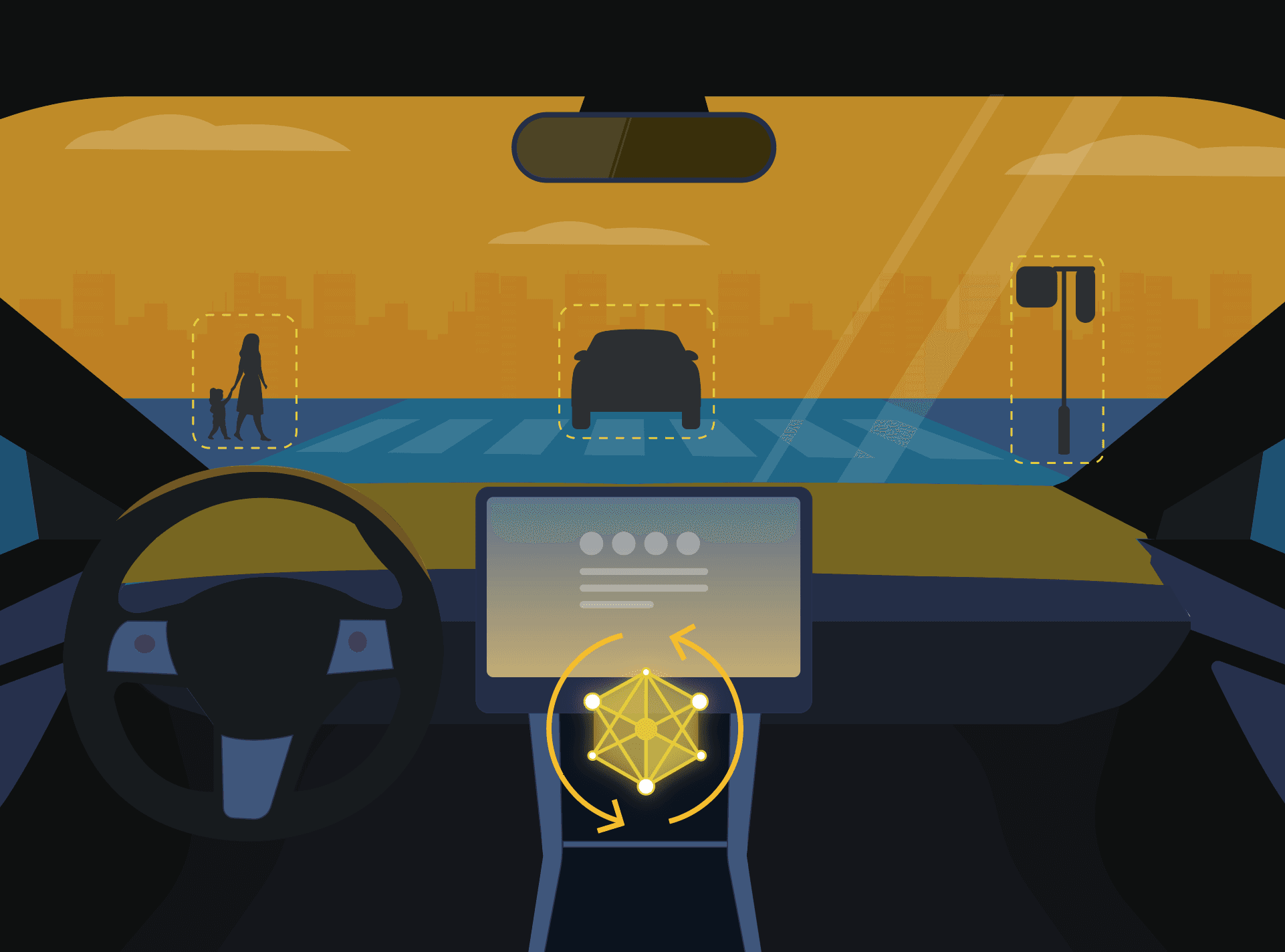
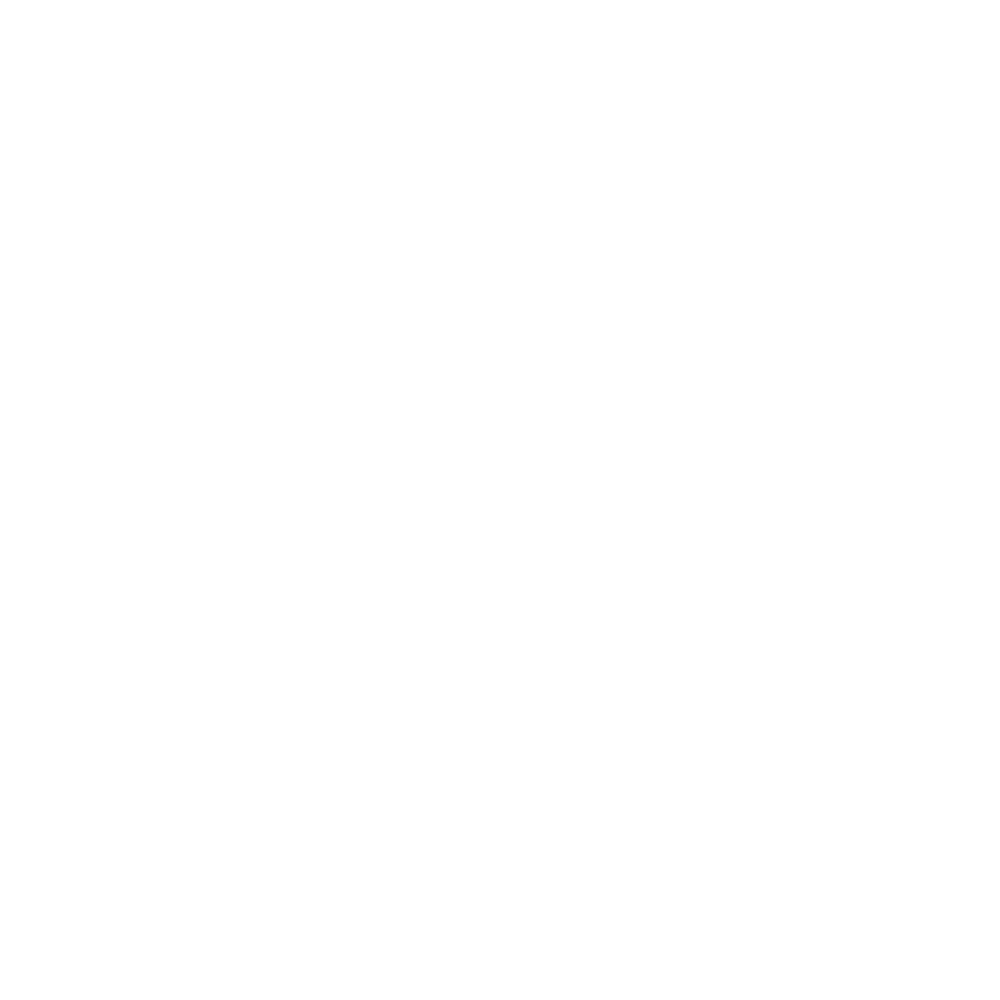
Federated Learning in Automotive
Empower automotive innovation through collaborative intelligence, securely advancing autonomous and connected vehicle technologies with Federated Learning
Federated Learning drives automotive innovation while enhancing data privacy
Federated Learning (FL) drives automotive innovation by harnessing collective intelligence from a wide range of vehicles, from personal cars to commercial electric vehicles (EV) trucks, all while ensuring data security. This collaborative approach, combining Federated Learning with Edge AI, unlocks numerous opportunities. Companies can tap into the collective knowledge of these vehicles while enabling real-time, on-device processing for immediate decision-making. This includes advancements in autonomous driving, enhanced predictive maintenance, personalized in-car user experiences, and optimized traffic management systems.
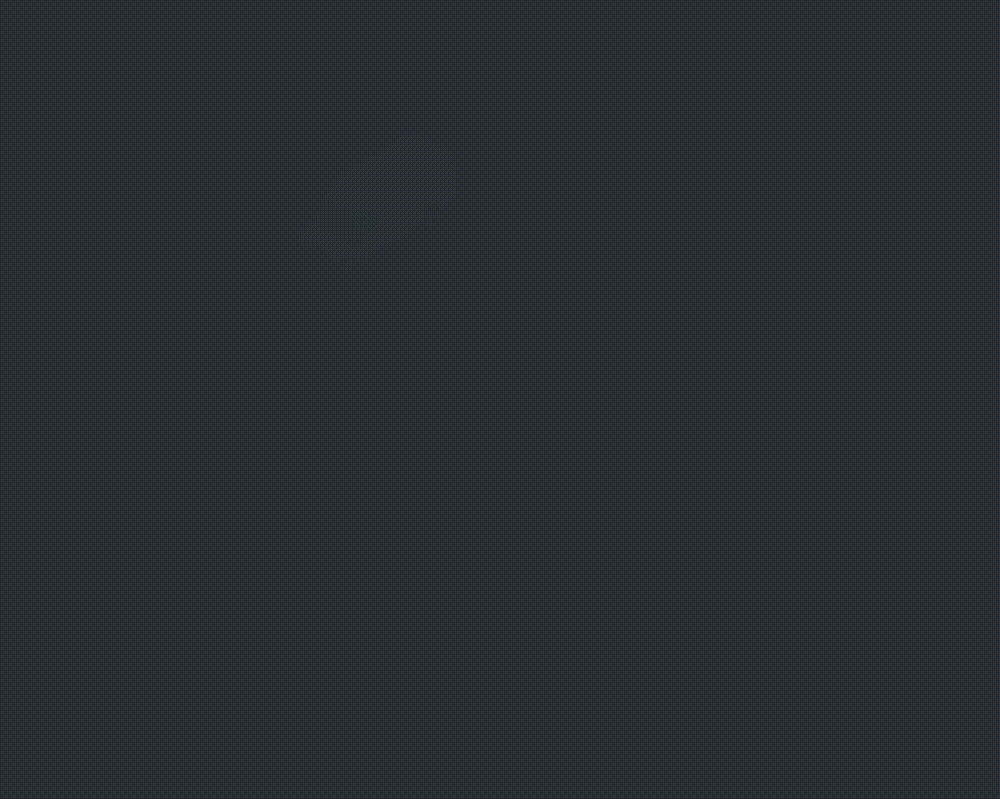
Driving Innovation Forward
- Personalized Models
Vehicle operators can develop personalized AI models based on their specific usage patterns and environments. For example, specialized AI Models can be trained to predict and respond to a driver’s specific behavior and customize the driving experience by adapting car settings to individual preferences.
- Privacy and Real-time Processing
Edge AI not only improves data privacy by keeping raw data local but also enables real-time processing critical for vehicle operations. For instance, EV trucks can make immediate decisions about power management without relying on cloud connectivity, enhancing efficiency and safety.
- Cost Efficiency
Since data is processed locally, costs are significantly reduced by:
- Minimizing large-scale data transfers to a central server, as only model updates are shared, which reduces reliance on cloud computing resources and centralized storage infrastructure.
- Enabling more frequent model updates and faster deployment, leading to quicker realization of benefits such as fuel efficiency and predictive maintenance.
- Implementing FL with efficient Edge AI hardware, like compact, energy-efficient processors, minimizes per-vehicle investment while maximizing computational capability and lowering operational costs for large fleets.
- Scalability
By leveraging data from multiple vehicles, we can create a more robust and accurate AI model that learns from a wide variety of scenarios and conditions. This reduces data bias and enhances the model’s ability to generalize and predict across diverse environments. Continuous updates ensure that the AI model stays current and effective.
Flower’s FL approach scales efficiently with fleet growth. As more vehicles contribute to the learning process, the global model becomes increasingly accurate and robust, all without a corresponding increase in central infrastructure costs.
Federated Learning’s Broad Applications in the Automotive Field
Explore other Applications Developed by the Community
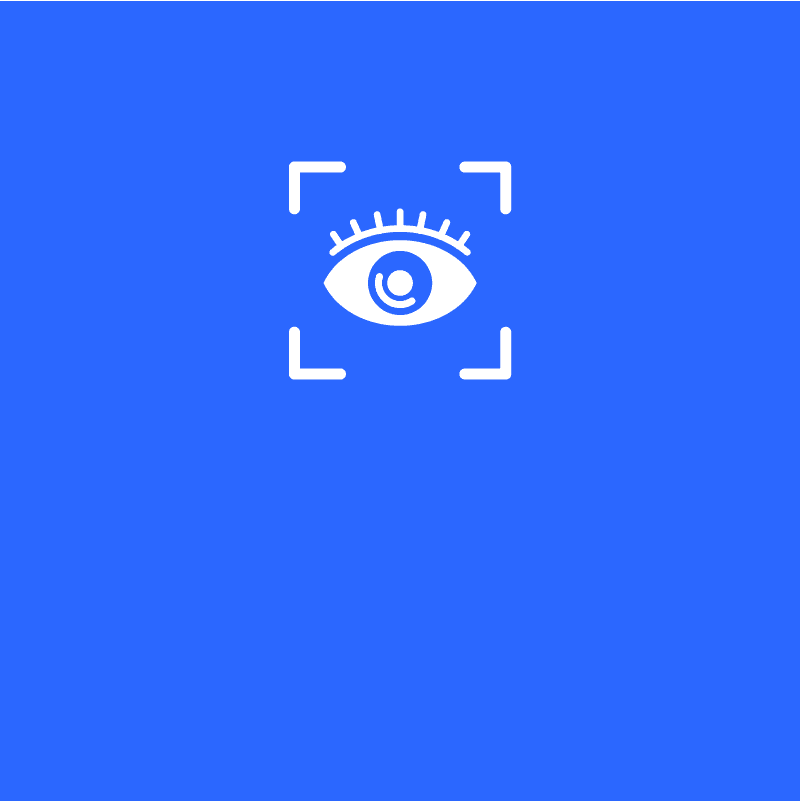
Federated Learning for Drowsiness Detection in Connected Vehicles
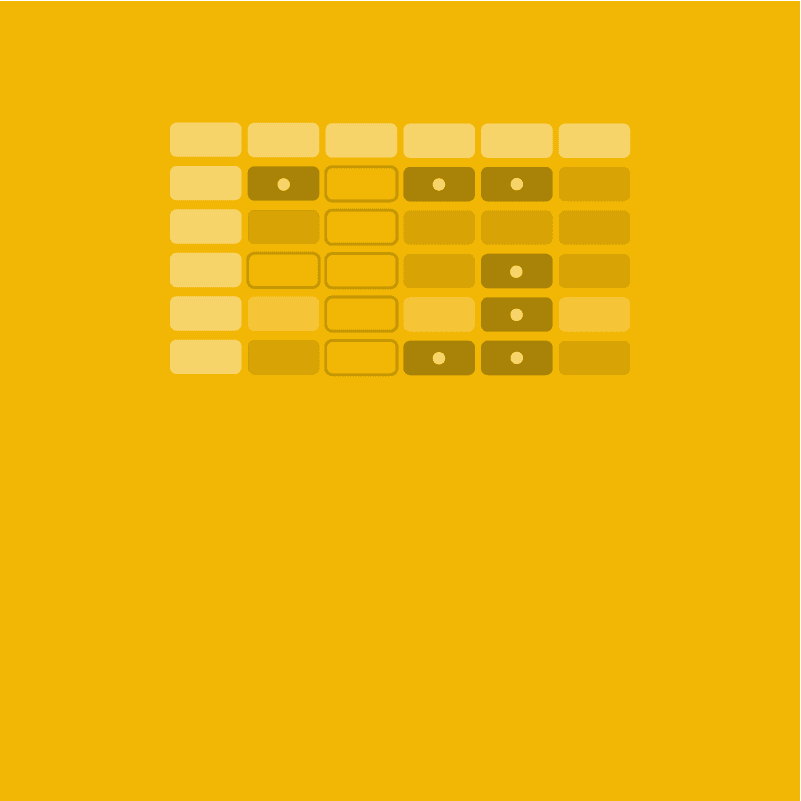
Federated Learning for Tabular Data using TabNet: A Vehicular Use-Case
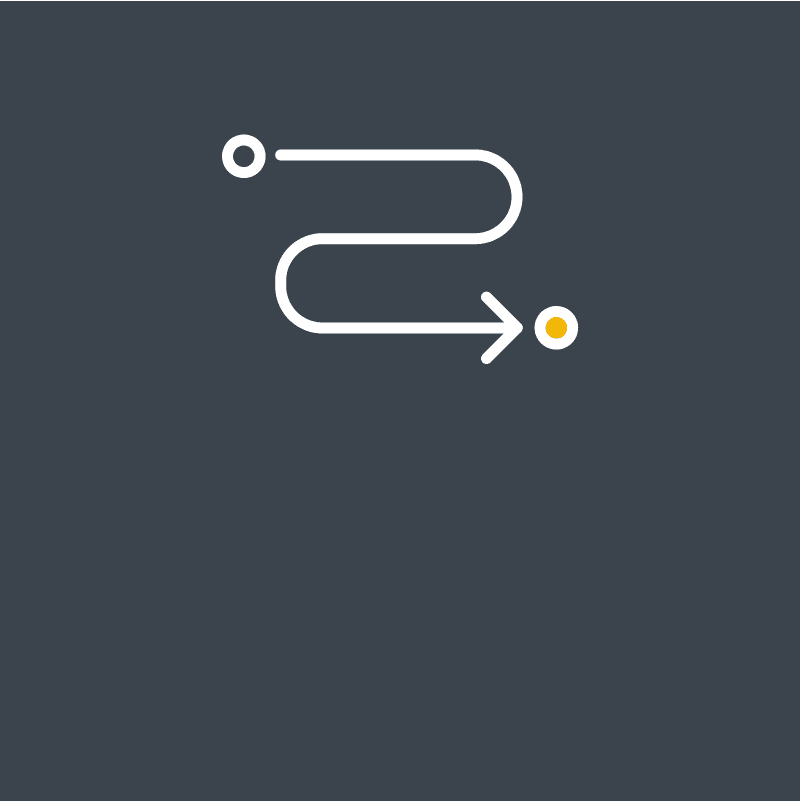
Towards Production-ready End-to-end Federated Learning for Automotive Applications
Experience the Future of Automotive with Federated Learning
Request a demo to explore how Federated Learning can revolutionize your project.