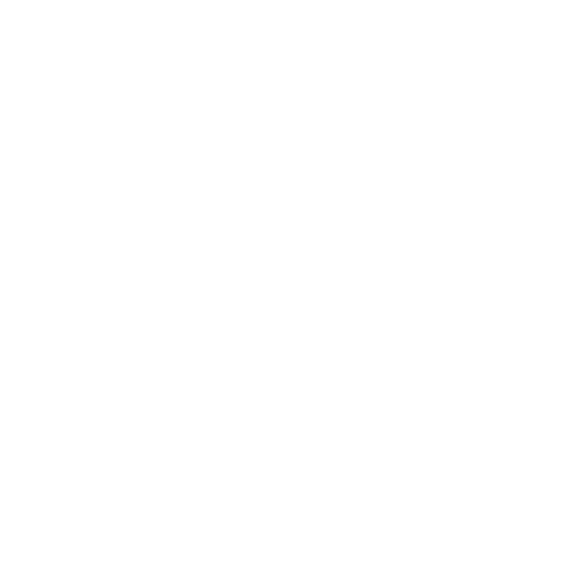
Federated AI in Finance
Enhancing security, compliance, privacy, and predictive accuracy in financial services through collaborative machine learning.
Federated Learning is Revolutionizing Finance
Federated Learning (FL) is revolutionizing finance by allowing institutions to collaboratively train machine learning models on decentralized data while enhancing privacy and compliance requirements. This approach enables financial organizations to use vast datasets from banks, insurers, and investment firms without sharing the underlying data. By aggregating insights from diverse sources, FL enhances model accuracy for risk assessment, fraud detection, and personalized services, all while supporting privacy standards and finance-specific regulations, making it an ideal solution for the finance industry.
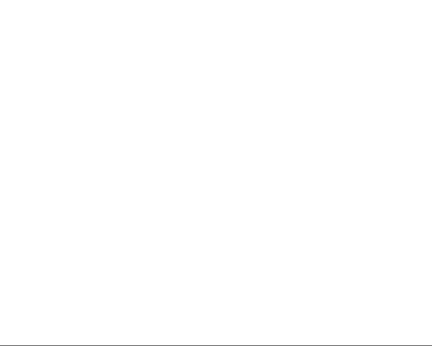
Challenges Faced in Finance
- Data Privacy and Security
Financial institutions handle highly sensitive data that must comply with strict privacy regulations. Federated learning helps to keep data localized, significantly reducing the risk of data breaches and ensuring compliance with GDPR, CCPA, and other regulations.
- Fraud Detection
Detecting fraudulent activities requires analyzing patterns across multiple data sources. Federated learning allows financial institutions to train models on a broader set of data from various entities, improving the detection and prevention of fraud with more comprehensive and accurate models.
- Risk Management
Accurate risk assessment models are crucial for financial stability. By using federated learning, financial institutions can develop more robust risk models by incorporating diverse datasets, enhancing the prediction of market trends, credit risks, and investment opportunities.
- Regulatory Compliance
Financial institutions must comply with regulations like AML, KYC, and Basel III, requiring robust monitoring and reporting. Federated learning enables decentralized data analysis, improving transaction monitoring and risk assessments while keeping sensitive data within regulatory guidelines.
Cross-Border Data
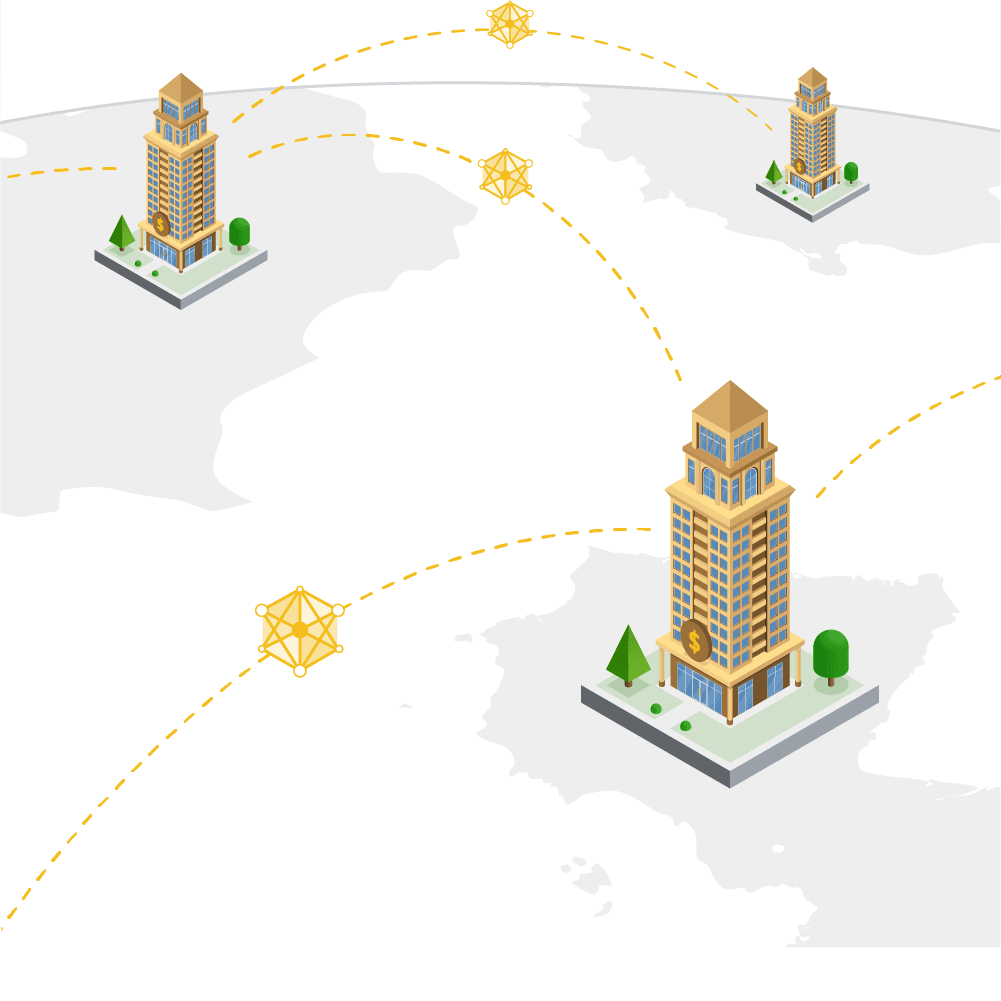
Financial institutions often operate across multiple jurisdictions, each with its own strict data privacy regulations, such as GDPR in Europe or CCPA in the United States. These regulations limit the ability to transfer sensitive customer data across borders for centralized analysis. As a result, financial organizations may struggle to leverage valuable data from different regions, hindering their ability to develop comprehensive models for tasks like risk assessment and fraud detection.
How Federated Learning solves this challenge: FL enables institutions to comply with data protection regulations by keeping data localized within its originating country or region. Instead of transferring raw data across borders, institutions train machine learning models locally on their data, sharing only model updates (such as parameters) with a central server. These updates are aggregated to improve a global model without ever exposing sensitive data. This ensures compliance with regional regulations while still benefiting from the insights of a broader, global dataset.
Cross-Organizational
Collaboration
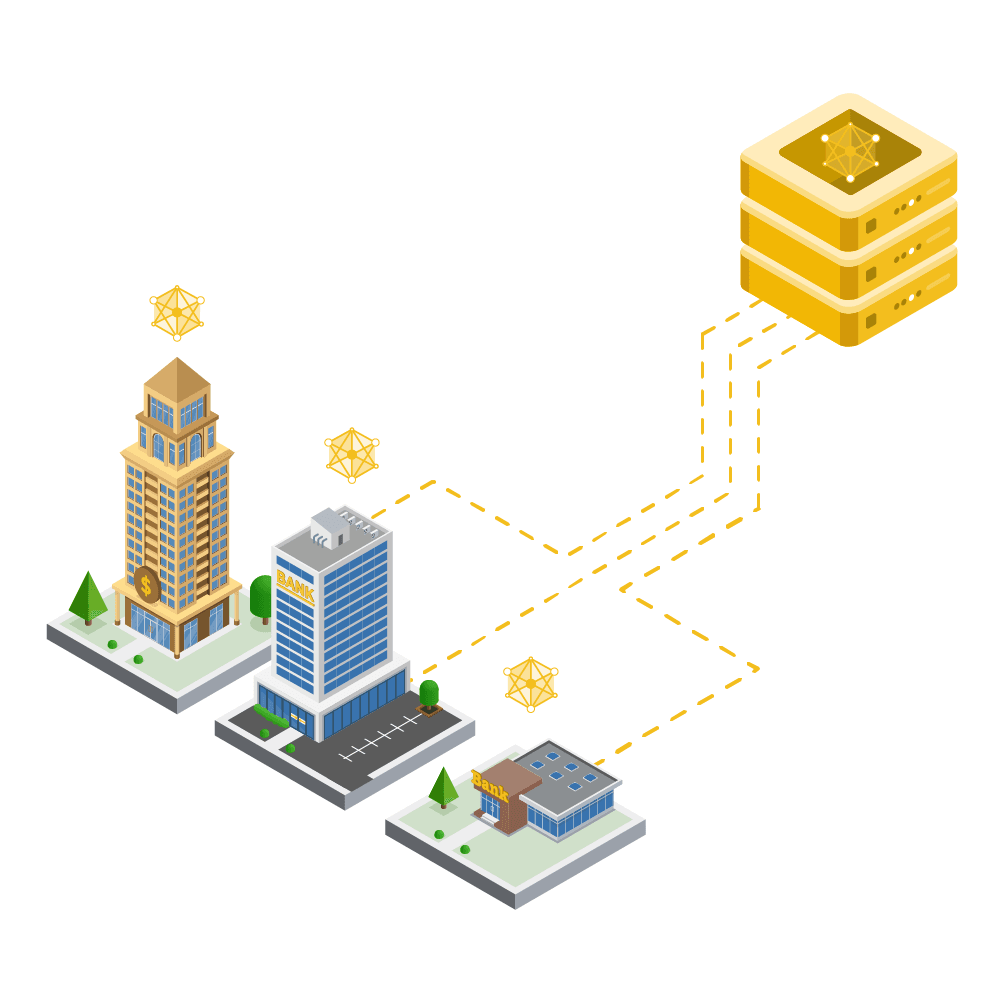
Financial organizations, such as banks, insurance companies, and investment firms, often work in silos, holding onto proprietary or sensitive data that they are reluctant to share with competitors. This lack of data sharing makes it difficult to collaborate on key issues like fraud detection, risk management, or personalized services, as these often require a broad range of data to identify patterns that one organization alone may not have access to.
How Federated Learning solves this challenge: FL enables secure, cross-organizational collaboration by allowing institutions to train models on their own data without sharing the underlying information. Instead, each organization trains a local model and shares only updates (like model parameters) with a central server, which aggregates the information to improve a global model. This method allows institutions to collaborate on shared challenges — such as developing better fraud detection systems or risk models — without compromising data privacy or competitive advantage.
Flower in production example.
Enhancing Banking Circle's Anti-Money Laundering System with Flower
“Flower was exactly the solution we were looking for.”
Banking Circle, a fully licensed next-generation payments bank, addresses the global banking needs of payments businesses, banks, and marketplaces. Through its API, the company offers fast, low-cost global payments and banking services by connecting to the world's clearing systems, enabling real-time liquidity movement for all major currencies securely and compliantly. Serving over 250 regulated businesses, Banking Circle processes more than 10% of Europe's B2C e-commerce flows and processed payments worth 558 billion euros in 2023. To address the time-consuming and costly nature of Anti-Money Laundering (AML), the company developed a transaction monitoring system that scans payments for suspicious behavior and pre-selects cases for manual analysis.
- Banking Circle is revolutionizing cross-border transactions by building a super-correspondent banking network, aiming to cut transaction times from five days to five seconds and costs from 50 euros to 50 cents. Tackling the intensive and costly process of Anti-Money Laundering (AML), they've developed an AI-powered transaction monitoring system that screens transactions for suspicious activity, reducing manual analysis time. This system, however, faces challenges in the US market due to differences in transaction types and data transfer difficulties.
- To overcome these challenges, Banking Circle employs Flower, a federated learning system, to train the AI model on European data without moving it across borders. This allows them to develop a model tailored to the US market, improving accuracy and efficiency over time. As the US model evolves, it feeds improvements back into the European system, benefiting both regions. Initial testing of this federated learning approach showed significant performance improvements, including a 65% increase in precision, a 25% increase in recall, and a 10% increase in accuracy, highlighting how federated learning can drive innovation in the finance industry by enabling transformative solutions.
FLAME
Federated Learning for Anti-Money laundering Enhancement
Experience the Future of Finance with Federated Learning
Request a demo to explore how Federated Learning enhances security, privacy, and predictive accuracy in financial services. Join the innovators transforming finance today!