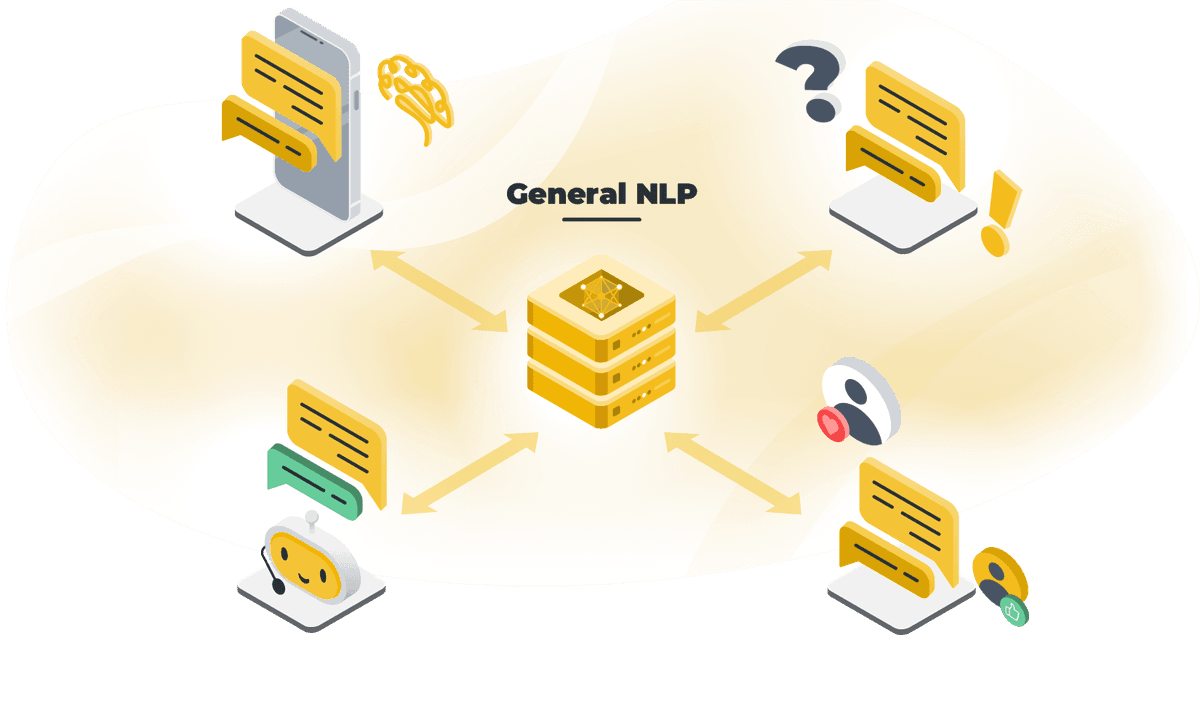
NLP LLM Leaderboard
Embrace Federated LLM Fine-Tuning and Secure Your Spot on the Leaderboard!
← Scroll →
In the realm of Natural Language Processing (NLP), developing models that can effectively understand and generate human language is foundational. Federated LLM fine-tuning of models trained on general NLP tasks is vital as it democratizes LLM training across a diverse set of downstream tasks while preserving data privacy. This approach enable that the fine-tuned language models are not only robust and generalizable across various linguistic contexts but also attuned to nuances and colloquialisms present in different datasets.